The Power of Annotated Image Datasets: Transforming Data Annotation for Business
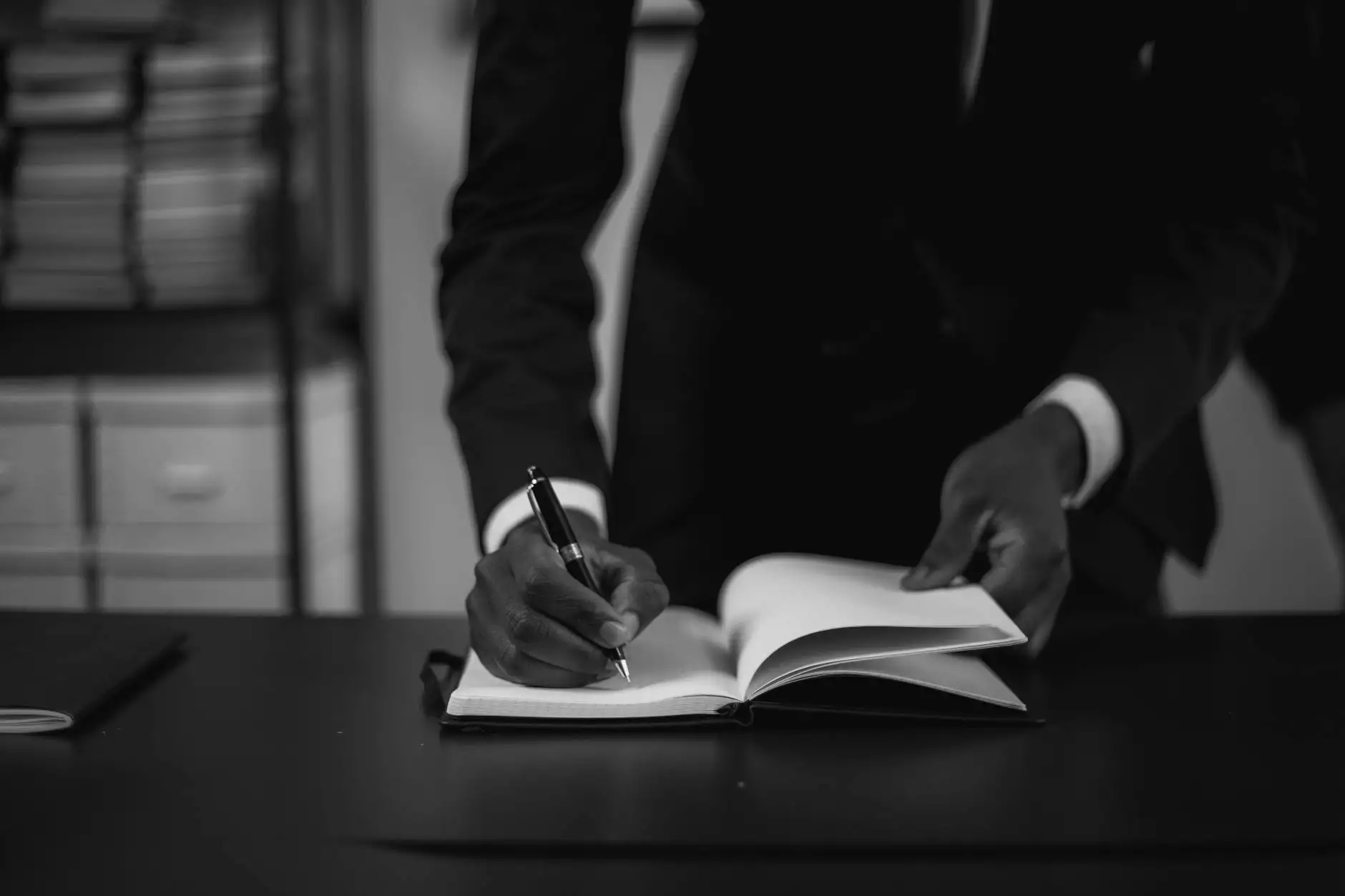
In today’s digital landscape, businesses are increasingly relying on data-driven decision-making to enhance their operations and offerings. One vital component of this ecosystem is the annotated image dataset, a powerful tool that plays a significant role in training artificial intelligence (AI) and machine learning models. This article delves into the essence of annotated image datasets, their relevance to data annotation tools and platforms, and how they can elevate business outcomes.
Understanding Annotated Image Datasets
An annotated image dataset is a collection of images that have been tagged with specific labels and contextual information. These annotations provide vital information to AI algorithms, enabling them to recognize patterns, objects, and features within images. The process of creating these datasets involves a mixture of human expertise and automated tools, ensuring high-quality outputs that are pivotal for successful machine learning applications.
The Importance of Data Annotation in AI
Data annotation is the backbone of any AI project. The effectiveness of an AI model is not only dependent on its algorithms but also on the quality of the data it is trained on. Here’s why annotated image datasets are so critical:
- Enhanced Learning: Models learn to identify and differentiate between various objects based on the tags given in the annotated images.
- Reduction of Bias: By using carefully annotated datasets, businesses can help mitigate bias in AI, leading to more equitable and applicable models.
- Increased Accuracy: Quality annotations lead to higher accuracy in predictions and classifications, which is crucial for applications in healthcare, autonomous driving, and more.
- Facilitated Testing and Validation: Annotated image datasets allow developers to rigorously test AI systems, ensuring their robustness before deployment.
Key Applications of Annotated Image Datasets
The applications of annotated image datasets span across various sectors. Here are some prominent use cases:
1. Healthcare
In the healthcare industry, annotated images are invaluable for training AI to recognize diseases in medical imaging. For instance, annotated x-rays or MRI scans help AI systems learn to identify tumors, fractures, or other abnormalities.
2. Autonomous Vehicles
Self-driving cars rely heavily on annotated image datasets to interpret their surroundings. Datasets containing annotated images of traffic signs, pedestrians, and other vehicles are crucial for training the perception systems of autonomous vehicles.
3. Retail and E-Commerce
Retailers utilize annotated datasets for facial recognition, sentiment analysis, and product categorization. For example, an annotated image dataset can help train a model to recognize different clothing styles for an online fashion store, enhancing customer experience through personalized recommendations.
4. Agriculture
In the agricultural domain, annotated image datasets enable the development of precision farming technologies. Drones equipped with cameras collect aerial images of crops, which are then annotated to assess plant health, detect diseases, and optimize harvesting strategies.
Features of a High-Quality Annotated Image Dataset
While businesses can benefit from any annotated image dataset, certain features can significantly enhance its utility:
- Diversity: A dataset should encompass a wide range of examples to cover various scenarios and conditions, reducing the risk of overfitting.
- Consistency: Annotations must be consistent across different images to ensure that AI models can generalize effectively.
- Detailed Annotations: The dataset should provide comprehensive annotations, including bounding boxes, labels, and segmentation maps, to facilitate different use cases.
- Scalability: The dataset should be designed to be scalable, allowing for continuous updates with new images and annotations over time.
Building an Annotated Image Dataset: Best Practices
For businesses looking to develop their own annotated image datasets, here are some best practices to consider:
1. Define Clear Objectives
Before embarking on the annotation process, it’s crucial to clearly define the objectives. This includes identifying the specific tasks the AI model will perform and the types of images required for training.
2. Choose the Right Annotation Tools
Utilizing a robust data annotation platform such as Keylabs.ai can significantly streamline the process. Advanced tools offer features like automated annotations, quality checks, and collaborative workspaces for teams.
3. Train Your Annotators
Human annotators play a critical role in the quality of the dataset. Providing training sessions can enhance their understanding of the specific annotation tasks, resulting in more accurate and reliable annotations.
4. Implement Quality Control Measures
Establishing quality control protocols is essential to ensure the integrity of the dataset. This could involve random checks of annotations, feedback loops, and using multiple annotators for the same set of images to compare results.
5. Regularly Update the Dataset
Given that technology and requirements evolve, regularly updating the dataset with new images and refreshed annotations ensures that the model remains relevant and accurate.
Challenges in Creating Annotated Image Datasets
While creating annotated image datasets provides numerous advantages, businesses may face challenges, including:
- Resource Intensive: The process of annotating images can be time-consuming and require significant manpower.
- Maintaining Quality: As the size of the dataset increases, maintaining a consistent quality of annotations becomes more challenging.
- Data Privacy Concerns: Businesses must navigate privacy laws and regulations, especially when involving images of individuals.
Leveraging Keylabs.ai for Data Annotation
For businesses seeking to harness the power of annotated image datasets, Keylabs.ai offers a comprehensive data annotation platform designed to streamline the entire process. Here’s how Keylabs.ai can help:
1. Scalability
Keylabs.ai provides the ability to scale your data annotation efforts as your business grows, allowing you to accommodate an increasing volume of images seamlessly.
2. Advanced Annotation Tools
The platform features innovative tools for image annotation, including automation options that enhance efficiency while maintaining quality.
3. Collaboration Features
With collaborative features, teams can work together in real-time, ensuring that annotations are accurate and consistent across the dataset.
Conclusion: The Future of Annotated Image Datasets in Business
The rise of AI and machine learning has ushered in a new era of data utilization for businesses. As organizations continue to embrace data-driven approaches, the significance of annotated image datasets cannot be overstated. By leveraging platforms like Keylabs.ai, businesses can efficiently create high-quality datasets that drive innovation and enhance decision-making processes. As we look towards the future, investing in advanced data annotation strategies will undoubtedly play a pivotal role in shaping the success and growth of businesses across various industries.
In summary, the combination of quality annotated image datasets and effective data annotation tools is essential for businesses aiming to leverage the full potential of AI. The capabilities offered by platforms such as Keylabs.ai not only simplify the data annotation process but also maximize the benefits gained from AI-driven insights, ultimately enhancing overall business performance.